- SPE Energy Stream
- Drilling Data Quality and Uncertainty: Pathways to Value
Drilling Data Quality and Uncertainty: Pathways to Value
Drilling oil and gas wells is a complex process involving many disciplines and stakeholders. This process occurs in an environment where information is often unknown, incomplete, erroneous or at least uncertain. Even so, there is little scientific auditing of drilling data quality and uncertainty during well construction. There are no placeholders in well construction databases to document uncertainty and its propagation. The SPE has formed a cross-disciplinary technical sub-committee to investigate how to describe and propagate drilling data quality and uncertainty. The sub-committee is a cooperation between the Drilling System Automation, Wellbore Positioning, and Drilling Uncertainty Prediction Technical Sections.
Current data stores record the results of processes, but have limited capabilities to record how multidisciplinary processes generate these results, or the relationship between the results. The webinar explores the use of semantic networks to capture these multidisciplinary data relationships.
A first example illustrates how semantic networks can capture the quality and uncertainty associated with Depth from a multidisciplinary perspective. Multi-layered graphs are useful to relate the processes utilized by the various subject matter experts. The subject of data quality and uncertainty does encompass quantitative evaluations, for which influence graphs or Bayesian networks are well suited.
Secondly, the misinterpretation of information by multiple parties may result in poor data quality. The many interpretations of equivalent mud weight (EMW) by various data programs, even when they use the same mathematical definition, illustrates this topic. The issue is due to the different assumptions made by the various programs about the parameters of the conversion from pressure to EMW. The solution is to ensure that software applications are transparent about which management they use for these parameters: they can then correctly interpret exchanged information, even though the pressure to EMW conversion utilizes different assumptions.
A third example illustrates the complexity of the proper management of data quality and uncertainty ingeo-pressure margin estimation. Geopressure margin estimation is an important aspect of well planning and execution. However, many sources of uncertainty affect the accuracy of the prognosis. These uncertainties are associated with data produced by many different disciplines. As subject matter experts tend to work in silos, they do not often share these uncertainties, and do not perform appropriate routine uncertainty propagation across disciplines. It is therefore important to understand the possible sources of uncertainty to better quantify the estimation of geopressures.
Software engineering teams across the industry can directly use the work of the Drilling Data Quality and Uncertainty Description sub-committee to account for data quality and uncertainty in their data models. Use of this work can also ensure that the exchange of information in done transparently and seamlessly. If standardized, it can help accelerate interoperability and avoid misinterpretation between software applications, which is another form of poor data quality. This work can substantially benefit other efforts through effective integration. Some of these integrated industry opportunities include the SPE sub-committees “Verification & Validation”, “Drilling and Wells Interoperability Standards”, and the “Wells Data Foundation” of the Open Subsurface Data Universe initiative.
SPE Webinars are FREE to members courtesy of the
Post Tags
Course Chapters
- 1Drilling Data Quality and Uncertainty: Pathways to Value - Chapter 1Media Type: Video
Credits
Earn credits by completing this course0.15 CEU credit1.5 PDH creditsSpeakers
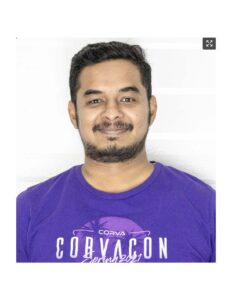